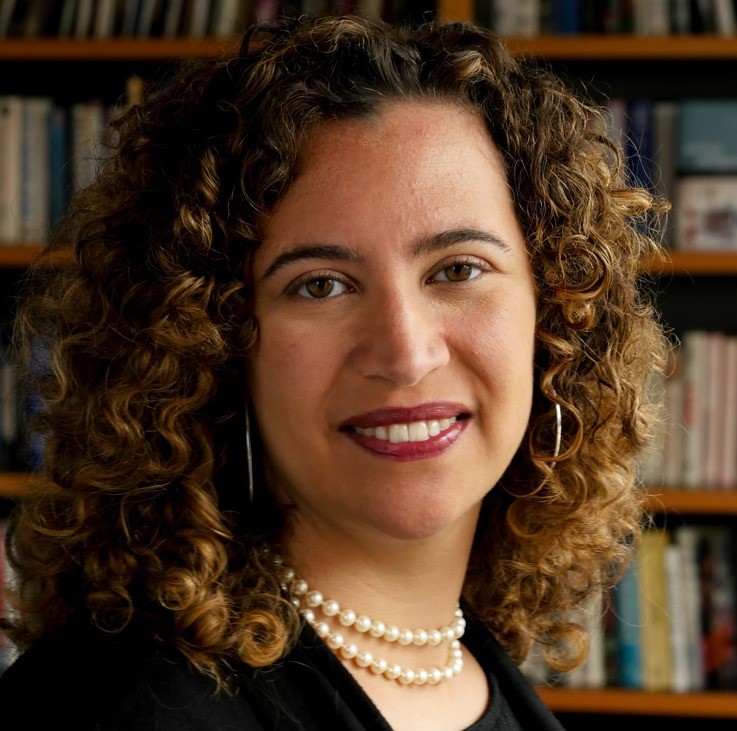
On March 27, 2024, Meredith Broussard, data journalist and associate professor at the Arthur L. Carter Journalism Institute of New York University, gave a presentation at the University of Michigan-Flint regarding the focus of her recent book More Than A Glitch: Confronting Race, Gender, and Ability Bias in Tech (2024). The book focuses on the ways in which artificial intelligence can be biased against people based on race, gender, and ability.
Broussard was previously a features editor at the Philadelphia Inquirer and has also worked as a software developer for AT&T Bell Labs and the MIT Media Lab. Her essays and features have appeared in The Atlantic and The New York Times, and also wrote an earlier book on artificial intelligence called “Artificial Unintelligence: How Computers Misunderstand The World”. She also currently serves as the Research Director at the NYU Alliance for Public Interest Technology, and on the advisory board for the Center for Critical Race and Digital Studies.
In her presentation, Broussard discussed some of the main topics in the new book. “It’s not magic, it’s math,” Broussard began her presentation, talking about how the kind of AI that we see in Hollywood films and television is not our current reality. AI is not sentient, it can’t think independently. AI doesn’t learn things the way a person might, it learns based on what’s fed to it by the people running the AI. She discusses how most generative AI systems are fed information scraped from various websites across the internet, and assimilate the bias of that content. Since humans are the ones writing the information being scraped and put into the AI, the AI “learning” process reflects the same biases on display in the content they “read”.
Broussard argues that AI systems discriminate by default. One example she offered was a recent investigation into a mortgage approval system using AI. Although the lender believed the system to be unbiased, the investigation revealed that the AI system was “48% more likely to deny borrowers of color as opposed to the white counterparts.” In some areas of the country, Broussard noted, that the percentage rose to more than 250%. She wanted to look into the mathematical and sociological reasons for why the system was biased, and found that it was biased because it was based on records of who had been given mortgages in the past, and made no adjustment for segregation and redlining. Thus, the societal bias was simply replicated in the AI.
She also talked about the bias in facial recognition using AI and how it is less accurate when examining the faces of people of color. She cites one study in the book that demonstrated that AI was from 10 to 100 times more likely to falsely identify Black or Asian people of color when compared to White people. It’s also more likely to work better on men than women, and she remarked that nonbinary, trans, or gender non-conforming people are often not recognized at all.
Other examples of unexamined bias in AI programming are detailed in her book. Broussard recounts how Amazon used an AI model to screen job applicants, only to find that the model discarded resumes submitted by women. Governments have used AI models to examine whether people were eligible for welfare, and found that the models discriminate based on gender, ethnicity, and immigration status. Even in medical care, the human biases are not examined, and lead to biased outcomes. The eGFR test tests kidney function, for example, but uses an AI risk model added a multiplier for Black black patients, and this meant that Black patients needed to be sicker before being put on a transplant list.
Broussard is not an optimist about the future of AI. She argues AI is not improving fast enough to address human inequality and bias that surrounds the creation of new AI models. Nevertheless she ended her talk on a note of vigilance. She encourages all of us to intervene when we recognize the real harms that emerge from AI, and the need to actively redesign our systems to create a more equitable world.